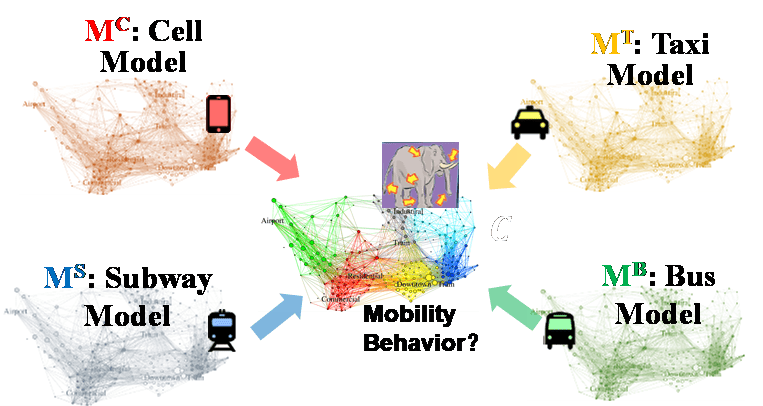
Intelligent transportation system data is multi-source with high variety. It covers several ways of residential mobility, including rail transit, route transit, floating cars and human walking trips. Different vehicles or human walking provides different data sources for ITS data and generally, the data formats from different sources are various (e.g., trajectory, traffic card readings). Meanwhile, ITS big data has the typical characteristics of massive volume, high dimensionality, sparsity, redundancy and noisy. All of these bring great challenges in data ETL processing for ITS big data. Intelligent Transportation Lab contributes to overcome such research difficulties. In our preliminary research, we have thoroughly analyzed the data properties of multi-source ITS big data and have proposed data processing and data fusion model utilizing spatial and temporal property of ITS big data.
In the preliminary research of data preprocessing, Intelligent Transportation Lab has developed a traffic sensing method that can recover traffic information with high accuracy at 85%, fully covering of a metropolis scaling of 2000 km2 with only 10 thousand floating taxi trajectory (i.e., 20% coverage of road segments). The traffic information in this research is represented by short-term average speed of each road segment calculated from the GPS measurements on each floating cars. By combining compressive sensing and spatial-temporal similarity analysis on city network, this research tackles the challenges of data sparsity and inconsistent recovery of different regions. In follow-up study, Intelligent Transportation Lab is focusing on introducing our latest technique on sparse representation (H. Xiong et al. 2019) to further transform traffic information into higher dimensional non-linear representation for more accurate traffic information recovery.
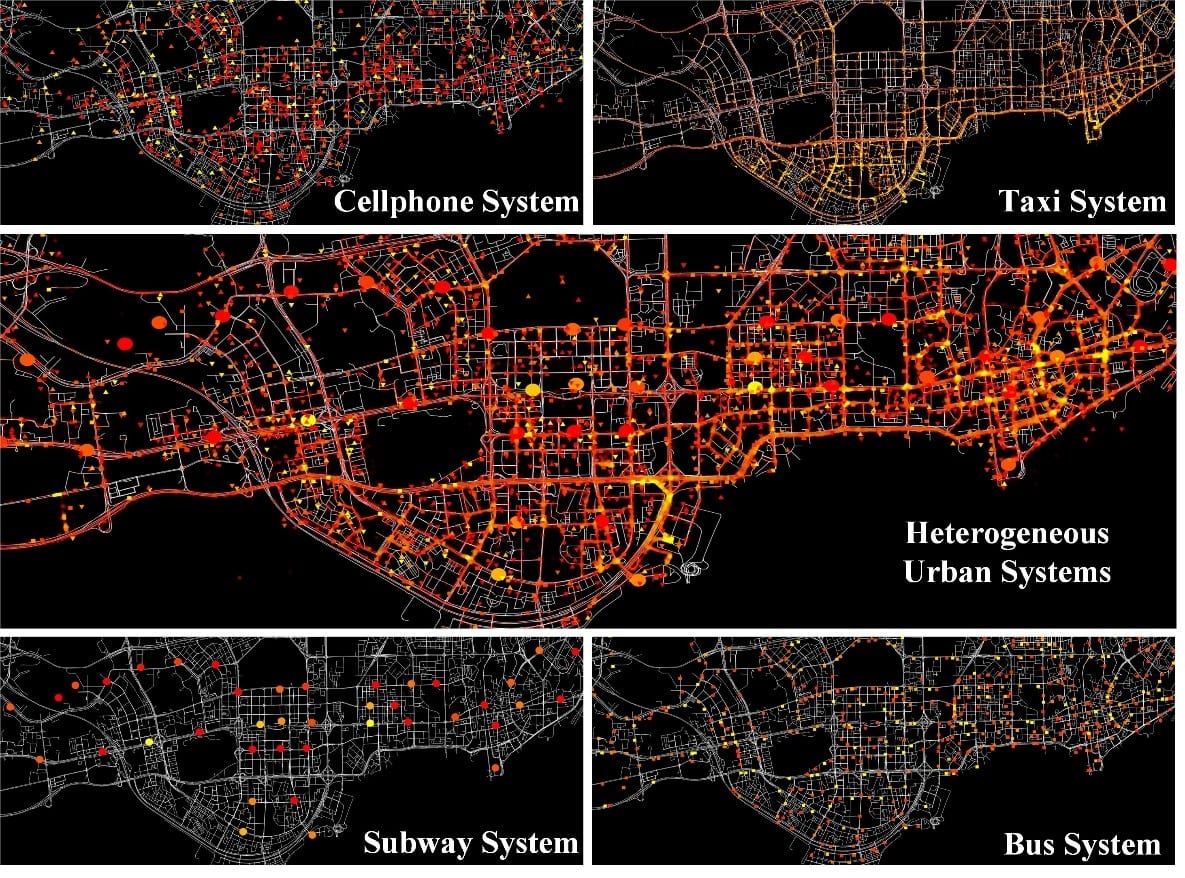
Intelligent Transportation Lab has proposed a cutting-edge and comprehensive framework for intelligent transportation research. This framework can integrate the spatial and temporal properties of city-scale traffic network into real-time traffic data fusion and it can also provide transportation recommendation based on data-driven analysis. This framework consists of three major components. The base component is real-time data feed layer which is built among multiple big data platforms (e.g., Hadoop, Spark) to support real-time data recovery, redundancy and noise removal. In this part, Intelligent Transportation Lab has proposed distributed scheduling system that can optimize based on data load balancing (Z. Yu et al. 2018). On top of the base component is the Mobility Abstraction Layer which defines residential human mobility and includes several offline/online inference algorithms on multi-source ITS big data (H. Zhang et al. 2018). Multi-source traffic data is to be fused into consistent mobility abstractions for the upper layer: application layer. Data-driven ITS applications can then be built inside the application layer by calling API to retrieve accurate mobility data from the last layer for detailed analysis. Demonstrated by experiments, our system can conclude and infer human mobility with more than 75% accuracy. The transportation recommendation system built upon can reduce up to 36% traveling cost for users. Related research also utilizes traffic analysis from this system, such as wireless charging lanes deployment problem (L. Yan et al. 2017).
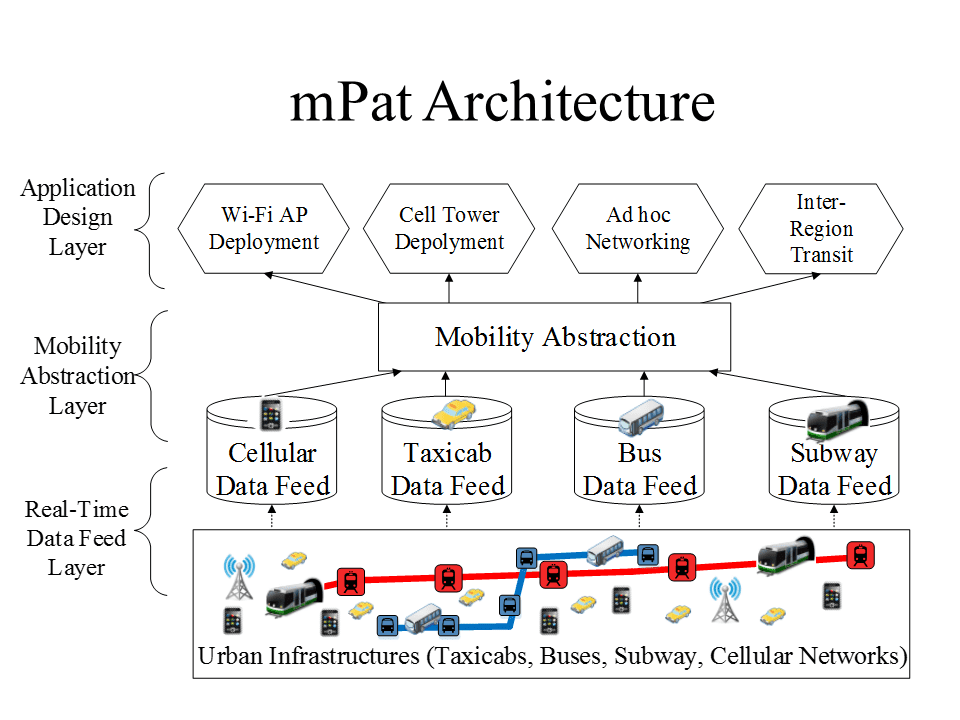
Intelligent Transportation Lab has also devoted into related research in data-driven ITS applications. One representative research project is to build shortest path index for large-scale network (Y. Li et al. 2017). In this research, we propose an efficient indexing schemes that utilizes network properties. It integrates efficient retrieval of network properties into effective indexing. Through experiments, this technique can enhance indexing time up to an order of magnitude.
Representative publication
- H. Xiong*, K. Wang*(equal), et al, SpHMC: Spectral Hamiltonian Monte Carlo, Proc. of AAAI, 2019.
- H. Zhang, et al, Urban-Scale Human Mobility Modeling With Multi-Source Urban Network Data. IEEE/ACM Transactions on Networking 26(2): 671-684 (2018).
- Z. Yu, et al, MIA: Metric importance analysis for big data workload characterization, IEEE Trans. on Parallel and Distributed Systems, 29(6):1371-1384, June 2018.
- L. Yan, et al. CatCharger: Deploying wireless charging lanes in a metropolitan road network through categorization and clustering of vehicle traffic. INFOCOM 2017:1-9.
- Li, Ye, Man Lung Yiu, and Ngai Meng Kou. “An experimental study on hub labeling based shortest path algorithms.” Proceedings of the VLDB Endowment 11.4 (2017): 445-457.